One of the biggest benefits of CQRS is the ability to implement multiple read models. Business rules and the domain model are safe, clean and isolated over in the write model. They are not getting in the way of view models, which can selectively pick the pieces they are interested in, freely reshape them, and do everything in a way that needs different kind of elegance and clarity as the domain model. The read models are all about query performance and convenience.
Put simply, CQRS is a practical implementation of what Pat Helland described in his paper on immutability: The truth is the log. The database is a cache of a subset of the log. Let’s have a look at some consequences of this approach.
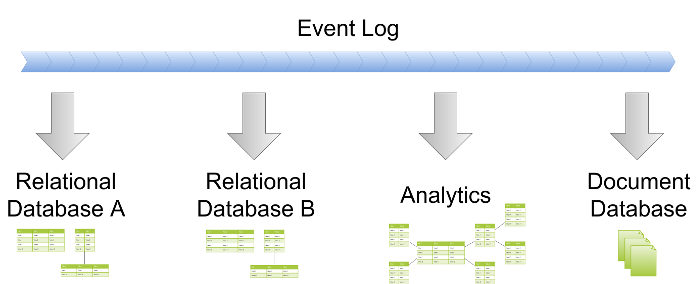
Persistent Models in Relational Databases
Perhaps the most obvious way to implement a read model is in a traditional SQL database. The technology has been around for decades, is really mature and battle-tested, and everyone is familiar with it.
However, in the CQRS world we can do things that would be problematic in a typical application database schema. Since we optimize for read convenience and performance, the data is very often denormalized. It can happen in a number of ways:
- There may be fields combining data from some other fields (e.g. a single text field with human-friendly street address).
- The same data may be present in more than one place, more than one table. For example it may make sense to have human-readable street address in a single column, but at the same time keep state and city in different columns (or table).
- Sometimes it also makes sense to keep multiple revisions or change history of an entity, not just the final version.
- Another good example of denormalized data is analytics (like OLAP cubes).
- The data does not have to be relational. You don’t need to map fields in a Java object to columns, or map a graph of objects to a number of tables. You can just serialize the whole thing (to JSON, XML, using native Java serialization etc.) and put it in a blob field.
Why Denormalize?
You may be wondering why one would be using a denormalized schema in the first place. The answer is: to do more computation ahead of time, while processing events and while no human is waiting for the answer. It means less computation at the moment a human is waiting for the answer.
The reason for this is that human time is expensive and getting more so, while computing power and storage is already extremely cheap and getting even cheaper. It is worth doing potentially a lot of computation in advance, to save a human a little bit of time.
How Much Denormalization?
The degree of denormalization depends mainly on performance and query complexity. Fully normalized schema has its benefits, but also many drawbacks. Numerous joins, calculations and filters quickly become tricky to write and maintain. They can also become performance nightmare, for example with joins between massive tables. Even if you’re not joining many thousands of rows, nontrivial calculations can keep the users waiting.
Denormalization can be used to prepare the answers for queries. If a query needs data that would normally live in several large tables, they can be combined once (in asynchronous projection), and then looked up in constant or logarithmic time when the users need it. It may even be possible to go to the extreme and precalculate responses to all common queries, eliminating the need for higher-level caching. In this case the view model is the cache.
It’s necessary to look for balance here, though. Overly aggressive denormalization can lead to poor maintainability related to code duplication, as well as increase the sheer volume of data (in terms of bytes).
Other Persistent Solutions
If the data doesn’t have to be relational, or if it can be denormalized, it may be a good idea to put it in a different kind of database. There is a wide range of NoSQL options to choose from, with the most obvious candidates being document and key-value stores.
We don’t have to stop there though – if the data could benefit from a graph database, there are no obstacles. Another great example of a view model are search indexes like Lucene.
Such stores often have their downsides. They may be trading off consistency for availability and performance. They may be very specialized or limited to particular models (graphs, documents, key-values etc.). It makes them challenging or even inapplicable as the primary persistence mechanism in a typical non-CQRS read/write model. However, they may be perfectly acceptable in a CQRS view model, and the advantages make the whole thing even more powerful.
In-Memory
Another idea we have been considering is in-memory models. Writing to and reading from disk is slow, and if the data fits in RAM, why not just keep it in memory, in ordinary data structures in your language of choice?
There are some challenges:
- If the event store is large enough, reading and consuming it may take a relatively long time and lots of resources. The limit may be farther away than it first seems, but it certainly is something that has to be carefully thought through.
- It needs to be transactional. It’s unacceptable for data to change while a query is reading it. You also may need to roll back, and that is far from trivial. It’s much easier in languages that support transactional memory or persistent data structures (like Clojure), and you would probably need a library with such functionality elsewhere.
These challenges could be solved by using persistent, transactional storage:
- When consuming domain events, update an in-memory model. Don’t touch the disk.
- Every now and then (e.g. every 1000 events or every minute) take a snapshot of that model and write it to some persistent storage.
- Let queries read from that persistent snapshot, possibly caching it in memory.
- After restarting the application or an error, continue consuming the events from the latest snapshot.
It’s getting close to persistent projections, but there are important differences. In this case persistence is only used for isolation and a way to resume from the savepoint after restart. Disk IO can happen asynchronously or less frequently, without slowing down the writer and queries.
Data Retention
Most queries are only interested in relatively recent data. Some may need a year or two, others may only be interested in the last week. With the source data safe on the domain side, the read models are free to keep as little as they need. It can have huge positive impact on their performance and storage requirements.
It’s also possible to have a number of models with identical schema but different data retention. Use the smaller data set as much as possible for best responsiveness. But still have the ability to fall back to a bigger data set for the occasional query about the faraway past, where longer response time is acceptable.
This approach can be combined with different granularity: Keep all the details for the last few weeks or months, and aggregate or narrow down for the longer time period.
Wrapping Up
NoSQL stores, analytics, search indexes, caches etc. are all very popular and useful tools, and very often they are used in a way resembling CQRS without acknowledging it. Whether they’re populated with triggers, messaging, polling or ETL, the end result is a new, specialized, read-only view on the data.
However, the more mature and the bigger the project, the harder it is to introduce such things. It may become prohibitively expensive, with missed opportunities eventually leading to many problems down the road.
It’s much, much easier if you have CQRS from the beginning. The domain model is kept safe and clean elsewhere, as is the ultimate source of data (like event store). The data is easily available for consumption (especially with event sourcing). All it takes to spin off a view model is plug in another consumer to the domain events.
The view models are very good candidates for innovation, too. It’s really easy to try various kinds of databases and programming languages, as well as different ways of solving problems with the same tools.
One thought on “Persistence in CQRS Read Models”
Comments are closed.